- Implementation of EO4Nature Use case - Forest Evaluation – Babadag Forest (BAIA Plot)
The vertical structure of forests is strongly correlated with aboveground biomass, productivity, and biodiversity. Therefore, accurate canopy height data is crucial for ecological research, particularly for biodiversity modeling and habitat quality assessment.
Given the environmental challenges posed by climate change, effectively managing biodiversity, biosafety, conservation, and ecological restoration requires the integration of Earth Observation (EO) satellite data into assessment and monitoring processes.
To estimate canopy height, we employ a probabilistic deep learning model that fuses GEDI data with Sentinel-2 imagery. The Canopy Height Model (CHM) utilizes an ensemble of convolutional neural networks (CNNs) to extract spectral and textural features from Sentinel-2 optical images, producing a high-resolution canopy height map. The model’s spatial and temporal resolution is further enhanced through sparse supervision using well-distributed reference observations from GEDI data.
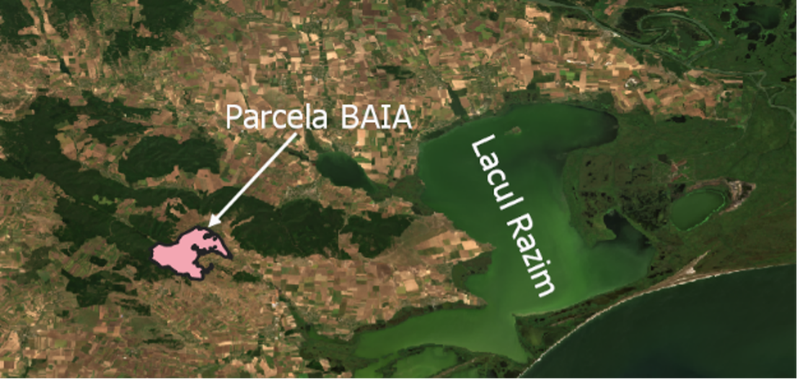
Our study focuses on the Baia forest lot within the Babadag Forest, Dobrogea. This area features a thermophilous deciduous forest, predominantly composed of Quercus petraea (sessile oak), with tree heights ranging from 10 to 40 meters.
We created a time series of 21 Sentinel-2 images, evenly capturing the phenological cycle of vegetation throughout 2020. For each acquisition, we extracted canopy height maps to analyze structural variations over time. Three of them are below:
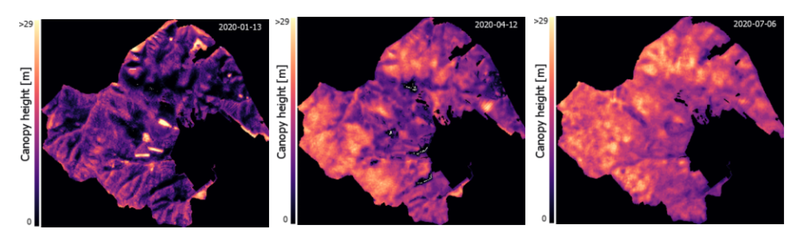
During the winter season, oaks shed their leaves, resulting in lower canopy heights. However, in areas with pine standswithin the Baia plot, higher canopy heights are expected during winter due to their evergreen nature. In the summer season, oaks develop a dense canopy, leading to increased estimated heights.